Wiki » History » Version 4
Version 3 (Ramon Martinez, 26 Jun 2014 09:36) → Version 4/23 (Ramon Martinez, 26 Jun 2014 09:50)
## Network models of V1
This project will be used to test implementations in PyNN (and eventually NeuroML) of published models of primary visual cortex (V1) based on spiking point neurons.
An initial focus will be on pubmed:14614078, but other models investigated will include pubmed:19477158 and pubmed:22681694.
This project is part of the [INCF Google Summer of Code 2014](http://incf.org/gsoc/2014).
### Troyer Model
Here I will describe breifly the implementation of Troyer et al (1998).
In order to run this model is necessary to first install [git](http://git-scm.com/) and [PyNN](http://neuralensemble.org/PyNN/) and the appropriate simulator.
After that you can clone directly from git using:
~~~
git clone https://github.com/OpenSourceBrain/V1NetworkModels.git
~~~
As the project stands at this moment the workflow can be described in two steps. First there is a script `produces_lgn_spikes.py` that creates the spike train for the cells in the Lateral Geniculate Nucleus (LGN). After the spikes are created they are stores in pickled format along with their respective positions to identify them downstream in the worflow. After we have the spikes train the file `lgn.py` uses the **PyNN's** SpikeSourceArray to create an LGN array with the spikes that we have produced in the other file. Using the stored positions we can, in the same file, create the thalamo-cortical connectivity using a Gabor-like sampling mechanism. The next step is to create the cortical-cortical connections with the correlations between cortical cells' receptive fields.
Next I describe the two first stages of the workflow, that is, the creation of the LGN spikes through the filtering of the stimuli and the thalamo-cortical connectivity
#### LGN - spikes
In brief, the Retina and the Thalamus part of the model can be represented by a spatio-temporal filter that, when convolved with the stimuli, will produce the firing rate of a given LGN cell. After that, we can use a non-homogeneous Poisson process to produce the corresponding spikes for each cell. We describe this in detail bellow.
##### Spatio-Temporal Receptive Field (STRF)
The file `kernel_functions.py` contains the code for creating the STRF. The details of the implementation are described in detail in the companion blog of this project [entry](http://neuralensemble.blogspot.fr/2014/06/gsoc-open-source-brain-retinal-filter-i.html). Down here we present a kernel produce with this classes. The time here runs from left to right and from up to down as usual text, so we can see how the spatial components of the filter change in time with this series of two dimensional maps.
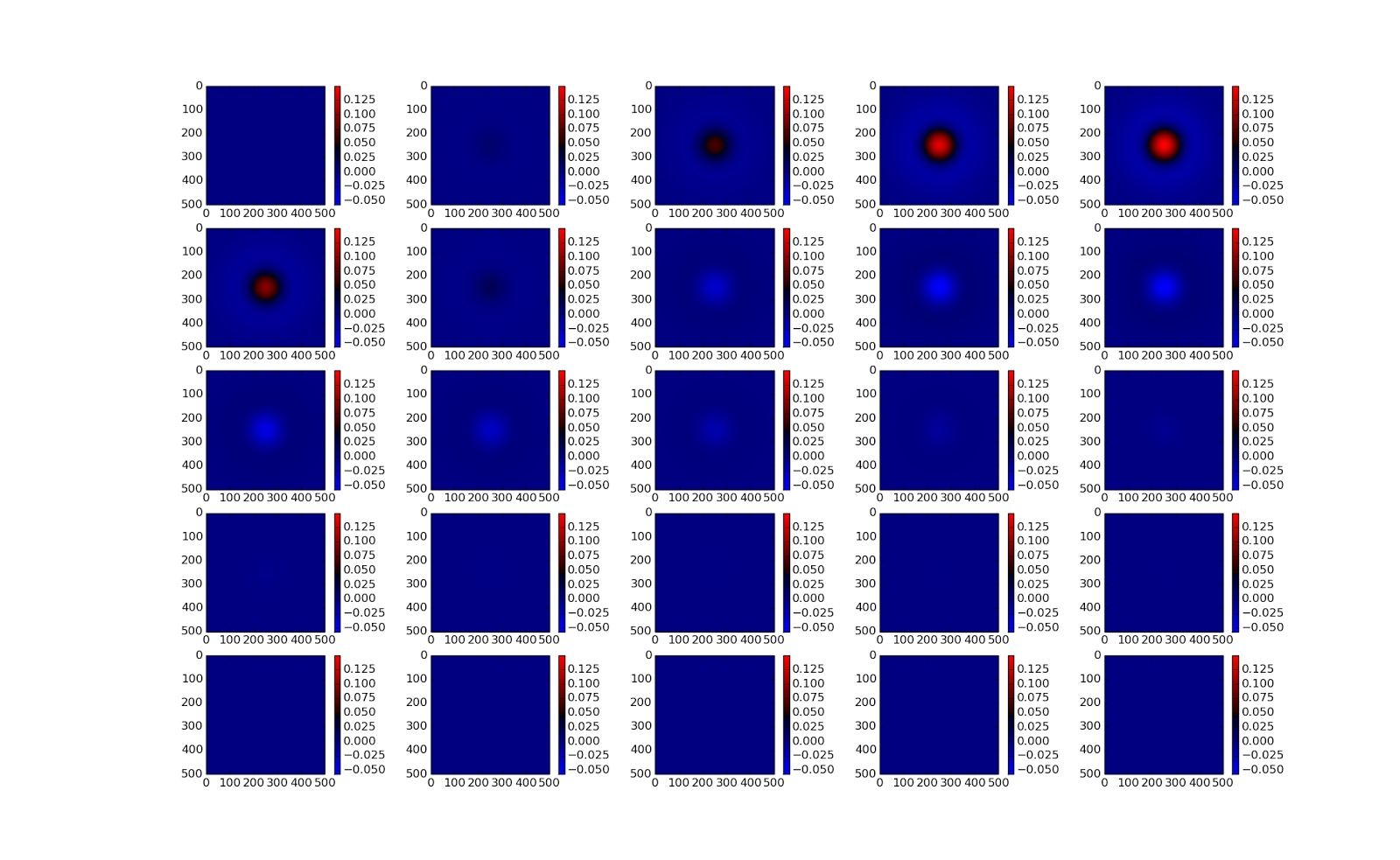
#### Stimuli
This project will be used to test implementations in PyNN (and eventually NeuroML) of published models of primary visual cortex (V1) based on spiking point neurons.
An initial focus will be on pubmed:14614078, but other models investigated will include pubmed:19477158 and pubmed:22681694.
This project is part of the [INCF Google Summer of Code 2014](http://incf.org/gsoc/2014).
### Troyer Model
Here I will describe breifly the implementation of Troyer et al (1998).
In order to run this model is necessary to first install [git](http://git-scm.com/) and [PyNN](http://neuralensemble.org/PyNN/) and the appropriate simulator.
After that you can clone directly from git using:
~~~
git clone https://github.com/OpenSourceBrain/V1NetworkModels.git
~~~
As the project stands at this moment the workflow can be described in two steps. First there is a script `produces_lgn_spikes.py` that creates the spike train for the cells in the Lateral Geniculate Nucleus (LGN). After the spikes are created they are stores in pickled format along with their respective positions to identify them downstream in the worflow. After we have the spikes train the file `lgn.py` uses the **PyNN's** SpikeSourceArray to create an LGN array with the spikes that we have produced in the other file. Using the stored positions we can, in the same file, create the thalamo-cortical connectivity using a Gabor-like sampling mechanism. The next step is to create the cortical-cortical connections with the correlations between cortical cells' receptive fields.
Next I describe the two first stages of the workflow, that is, the creation of the LGN spikes through the filtering of the stimuli and the thalamo-cortical connectivity
#### LGN - spikes
In brief, the Retina and the Thalamus part of the model can be represented by a spatio-temporal filter that, when convolved with the stimuli, will produce the firing rate of a given LGN cell. After that, we can use a non-homogeneous Poisson process to produce the corresponding spikes for each cell. We describe this in detail bellow.
##### Spatio-Temporal Receptive Field (STRF)
The file `kernel_functions.py` contains the code for creating the STRF. The details of the implementation are described in detail in the companion blog of this project [entry](http://neuralensemble.blogspot.fr/2014/06/gsoc-open-source-brain-retinal-filter-i.html). Down here we present a kernel produce with this classes. The time here runs from left to right and from up to down as usual text, so we can see how the spatial components of the filter change in time with this series of two dimensional maps.
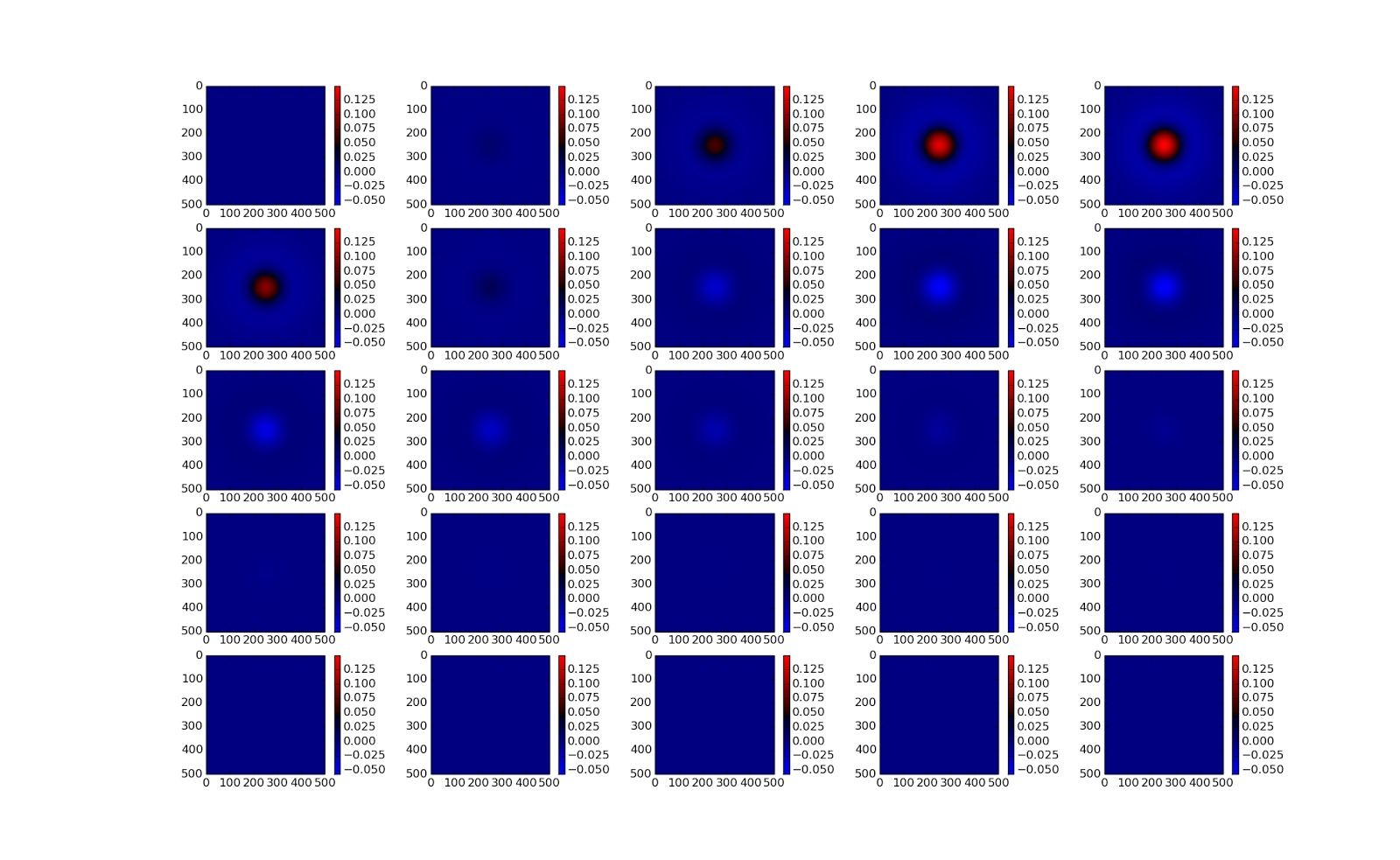
#### Stimuli